Faculty Spotlight: David Lary
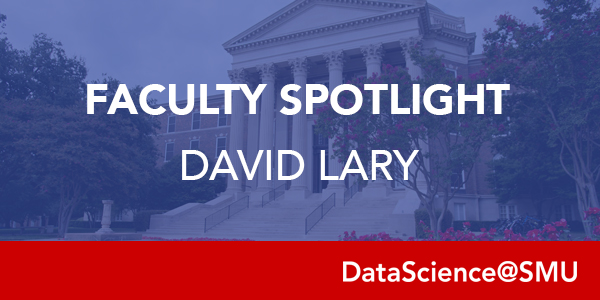
An accomplished data scientist, SMU’s Professor David Lary pioneered the use of machine learning, and his work appears in more than 150 publications. Now teaching future data scientists about Machine Learning in the online Master of Science in Data Science program at SMU, Lary spoke with us about his passion for data-driven service, his extensive background spanning decades, and the benefits as well as advancements in machine learning.
Tell us a little bit about yourself, your background, and what drew you to the world of data science.
My passion for data-driven service has spanned three decades. From 1986–91, I developed the first global 3-D model of ozone depletion just after the discovery of the stratospheric ozone hole by my colleague at Cambridge, Joe Farman. Once I had written the global 3-D model of ozone depletion, which was a plug-in for a weather forecasting model, I wanted to see how good the predictions were by comparing its results to actual data from as many sources as possible – sources measuring the composition of the atmosphere, including satellite instruments, airborne instruments, balloon instruments, and ground-based instruments. Then in 1995, with a partner from the European Centre for Medium-Range Weather Forecasts, I pioneered the first application of data assimilation for atmospheric chemistry. Data assimilation in meteorology had just led to a quantum leap in the accuracy of weather forecasts, and we wanted to extend this to forecasting air quality. It is gratifying that this contribution was acknowledged internationally by the British Royal Society through a 10-year fellowship, by being invited to join NASA as their first distinguished Goddard Fellow in Earth Science (2001–10), by six NASA technology and special service awards, and by Israel through an Alon Fellowship. Today NASA, NOAA, and the EPA, as well as several other international agencies, all use chemical data assimilation in the preparation of real-time environmental forecasts.
As part of this work, I was involved in the validation of several satellite instruments. This highlighted the ever-present issue of inter-instrument bias. Quite by chance, I came across machine learning about 20 years ago and found, to my delight, that it could be used to effectively account/correct for inter-instrument bias. Then I was curious to see what else it could do, and with each new application, it proved even more useful. I was hooked. This changed the direction of my work, and over the last 20 years, I have pioneered the use of machine learning coupled with massive remote sensing and other data sets for societal benefit. This body of work has led to more than 150 publications so far, with a total of nearly 2,700 citations in the peer-reviewed literature.
What does it mean to “teach a machine”? What is your approach to teaching machine learning and why is it important to incorporate machine learning into data science programs?
I like to think of machine learning in the following way: Just as humans learn by example, machine-learning algorithms can learn by example. It allows us to both learn from the past to inform the future and to give our data a voice, for example, through the application of unsupervised classification. It is critical to remember that there are two equally important components for the successful application of machine learning – a good algorithm and a comprehensive set of training examples that span as much of the system of interest’s parameter space as possible.
The approach that I find most effective is to start with a portfolio of case studies to give an overview of the type of things machine learning can do, then to introduce each area of machine learning and a description of how to test its performance, followed by hands-on exercises. The learning through doing is critical.
Where does your interest in machine learning stem from? What aspects of it excite you most?
I find it exciting that in machine learning we have a set of tools that allows us to learn by example, and then actually use these empirical models for practical applications. Further, the tools often allow us to gain insights into the data, rank the relative importance of the features, and actually allow us to make a difference for issues where we do not have a perfect theory.
You have been involved in research surrounding atmospheric composition. How has machine learning aided your research?
The machine learning allowed us to deal with some otherwise insurmountable issues. This is what first impressed me about machine learning 20 years ago, and I have not been disappointed ever since.
How can machines, arguably more advanced than people, be taught by people?
The various machine-learning algorithms are really an implementation of the scientific method, where we generate hypotheses based on the examples contained in the data and then test them to see if they hold up (generalize). So the algorithms can be run on a variety of platforms, from cheap single board computers, to mobile phones, to regular computers, and really high-end systems. The larger, more powerful high-performance computing can typically deal with larger problems, but the approach is the same.
In 2016, Google identified machine learning as “the future” of data. How will the continued development of machine learning affect future employment opportunities?
It will both open up exciting new opportunities while others will be rendered obsolete.
Which industries stand to benefit from machine learning advancements? Where do you see opportunities for machine learning to expand over the next five years?
Machine learning is making a difference in so many areas. Things started relatively slowly with applications such as credit scores, spam filters, character recognition, and electrical load forecasting. A major step forward was the ability to more effectively process images and recognize objects and more recently, video, using approaches such as deep learning. Then it turned out that these algorithms were also useful for a wide variety of applications such as language translation, even artistic endeavors such as musical composition in various styles, image hallucination, even document creation. Several of these areas become key aspects of control systems, such as for self-driving cars and aerial vehicle control. Most recently, approaches where the algorithms can teach themselves are emerging. So in my opinion, they will and are having an impact across the board, and the rate of progress is likely to accelerate dramatically.
Last updated November 2017